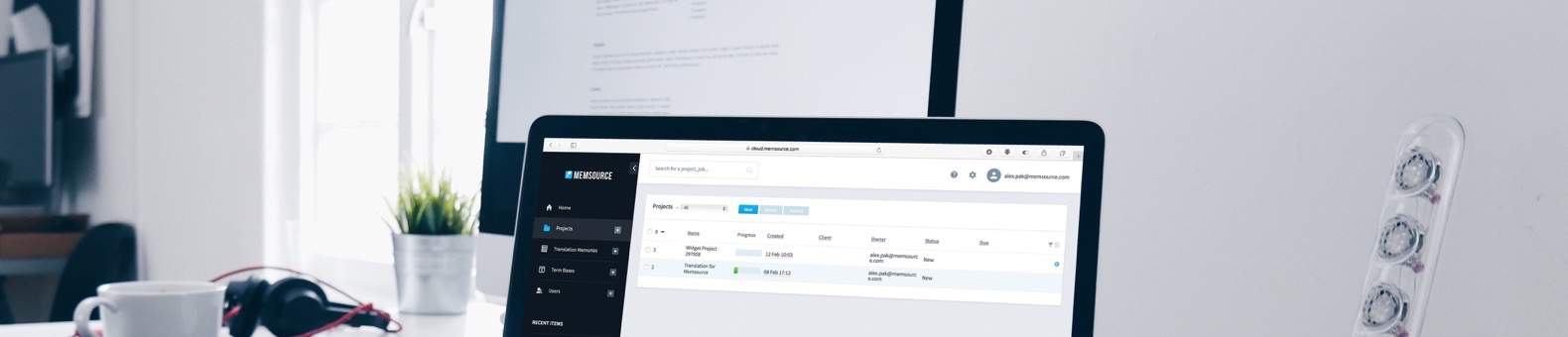
Blog
Contents
Machine Translation Report
What is the optimal MT Engine for you? Find out in the latest MT Report by Memsource.
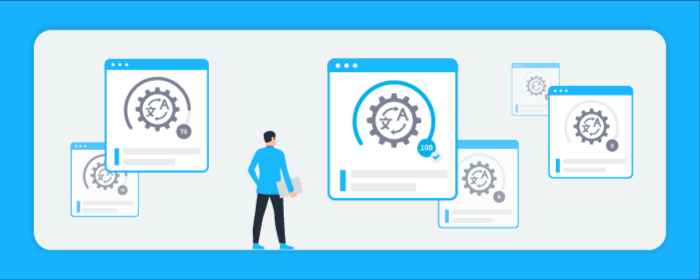
Machine Translation (MT) has taken tremendous strides in the past decade to improve its quality and make itself an essential part of translation workflows. Yet taking full advantage of MT can be tricky for both new and existing users who are unsure of how to choose the right engine. We’re giving you a breakdown on MT engine quality and how to choose the optimal engine for your content.
A complete newcomer to Machine Translation? Check out our Beginner’s Guide to Machine Translation.
Start your (machine translation) engines
Whether you are starting with MT or already leveraging it in your translations, the single most important factor is your MT engine.
Today there is an enormous number and variety of MT engines to choose from. The MT landscape is also continuously changing, with the constant release of new engines and the ongoing improvements of existing ones. Picking out the best performing engine can be a complicated and frustrating process.
It helps to think of the big picture. The main advantages of using MT are time and cost savings: the speed of translation is effectively instantaneous and the cost minuscule when compared to human translation. This is generally true of all MT engines available today.
This leaves only one major stumbling block: the quality of the MT output. This is perhaps the most important variable to consider when managing MT workflows, as poor output can jeopardize the gains in time and cost.
About machine translation quality
Recent developments in MT, key among them the wholesale transition from statistical machine translation to neural machine translation , have dramatically improved the base level quality of MT output. Our own internal data suggests that since 2017, the likelihood of getting a near-perfect segment, requiring minimal post-editing, has nearly doubled. The most commonly used engines today are likely to produce passable translations that can convey the meaning, if not exactly the nuance of the original text.
The trust you place in the quality of MT is largely dependent on the size and importance of your task. A student hoping to quickly translate a few lines of homework before his language class (shame on you) doesn’t have to be especially picky: all of the major MT engines used today are likely to produce a passable translation. Errors are more likely to happen due to an ambiguous source text, rather than a poor MT engine. But if you are looking to translate your life motto into French or Chinese for an interesting tattoo, you might want to have it double checked by a native speaker. The internet has no shortage of pictures of bad tattoos, testament to people who put too much trust in their MT engines.
Things change with scale. For a large enterprise a “passable” translation may not be good enough. With a growing volume of translations simple errors can start to add up and the likelihood of catastrophic errors is proportionately increased, ultimately requiring more extensive (and expensive) human review and post-editing. Cents become dollars and the workflows start to slow down.
An increase in scale can also reveal positives. The more you translate, the more likely you are to see differences between MT engines that you might not notice when focusing on smaller samples. Incremental differences will start to add up. Some engines will perform better, and using the right engine could result in comparative increases in quality and savings. Choosing the best performing engine is important.
See how different MT engines stack up in our Machine Translation Report.
Machine translation engine types
When choosing an MT engine you can opt for either a generic engine, such as Amazon Translate, Google Translate, or Microsoft Translator, or for a custom engine. Both types of engine rely on past translation data to produce their results.
Custom engines are trained using data that you provide them with to help refine their output. Successful past translations are used to guide the engine, making it more likely to produce the kind of translations that you are used to. Travel and hospitality content, for example, is especially suitable for custom engine training. Hotel listings or user reviews often share similar characteristics, and the sheer amount of content available makes engine training both possible and desirable.
This specificity is the greatest advantage of a custom engine, but also its main drawback. By focusing on specific types of content, out-of-domain performance is likely to be worse. Your engine, trained on hotel descriptions and reviews, may perform poorly translating news articles.
Custom engines are generally more expensive to set-up and maintain. They are well-suited for businesses that handle large volumes of copy that are quite similar in style and content and are able to justify the slightly higher costs involved.
Generic engines are the best options for most users, as the set up is quick and the costs significantly lower than those of customizable engines. Choosing one engine over another may be a slightly more complicated process if you place a premium on quality.
Evaluate or estimate machine translation quality?
When choosing an engine it is always a good idea to evaluate the MT output quality to know if you will be getting your money’s worth. Many MT users carry out extensive evaluations of all available options before they commit to an engine. The industry has adopted a number of quality metrics to help standardize the process.
We generally can distinguish between quality evaluation and quality estimation.
Quality evaluation relies on evaluating the quality of MT output, usually in reference to a human translation of the same source text. While most readers can easily determine which translation is more ‘natural’, a purely subjective evaluation will not be able to effectively evaluate at scale.
One method of evaluation is to rely on the evaluation of bilingual experts, who rate the quality of the MT output and the output of professional translators in a blind test. In the past, this method has been used to make some bold claims about the rising quality of MT, but it does have some significant limitations.
Primarily it is a question of cost: carrying out this test requires human translators and human evaluators. To get an accurate evaluation you may need to invest significant resources in the test. There are also concerns about the subjectivity inherent in any evaluation; studies have shown that professional translators are more likely to give higher marks for human translation, as opposed to non-professional linguists. Similarly, evaluation at segment level is more likely to reflect favorably on MT, as opposed to evaluating the segment in context of the whole article.
An alternative is to rely on computer algorithms to evaluate high volumes of translation quickly to produce an objective numerical score. This score is produced by an automated comparison of the MT output with a reference translation. The exact variables involved in the calculation differ from algorithm to algorithm, but generally speaking the closer the MT output is to the reference translation, the higher the score.
There is an enormous variety of different algorithms, the most commonly used today include:
- BLEU (BiLingual Evaluation Understudy)
- ROUGE (Recall-Oriented Understudy for Gisting Evaluation)
- METEOR (Metric for Evaluation of Translation with Explicit ORdering)
Each of these algorithms takes a different approach to measuring how “similar” the MT output is to the reference translation, and their relative advantages and disadvantages are a debate in themselves.
Generally speaking quality evaluation is an effective way of evaluating output that gives the user a lot of control over the process and a reliable result that allows effective comparisons between engines. However, the need to have human translated texts as a reference point and the process of setting up the evaluation itself make this a relatively slow and expensive method. An added malus is that these evaluations effectively produce ‘snapshots’ of a given point in time. Most MT engines today improve rapidly over time, so yesterday’s results might not be true today.
Quality estimation works differently. Rather than evaluating the output of an MT engine, it instead analyzes the source text you wish to translate and, based on certain criteria, predicts how good the translation might be.
To take an example that’s close to home, Memsource has developed a form of quality estimation, known as Machine Translation Quality Estimation (MTQE). No reference translation is needed, only the source text, as the estimation is powered by past performance data. The “quality” itself is estimated as the need to make edits to the output produced by the engine. With MTQE this is expressed as a percentage assigned to specific segments of the translation: a score of 100% would suggest that this particular segment is perfect and requires no edits, a score of 75% suggests that there might be some room for improvement. Though the estimation is made on a granular level for each segment, cumulatively the scores can give you an idea of how well the engine performs. One of the benefits of quality estimation is that it is a dynamic process that is continuously improving based on user feedback and improving its results. It is not ‘static.’
Now whichever method you choose will provide you with a sense of how the different engines will perform and which might be the ideal candidate for your machine translation needs.
More than one machine translation engine?
It is also important to note that you do not have to commit to a single engine. Most translation management software enables its users to switch relatively quickly from engine to engine. You might find that engine A is suitable for a certain language pair, while engine B is better suited for translating specific kinds of content. If you commit yourself to engine A or B exclusively, you will lose out on the quality gains achieved by the other engine in specific areas.
At Memsource we have developed Memsource Translate, a unique machine translation management solution that allows you to conveniently leverage multiple engines for the best possible translations. Our AI-powered algorithm automatically selects the best performing MT engine for your content, based on the language pair and content type of your document. Data on engine performance is collected in real-time and used to continuously update the algorithm’s recommendations. Memsource Translate comes with three fully managed engines and allows users to add their own, including customizable engines. The process of engine management and testing becomes automated, helping both newcomers to MT and existing users to optimize their workflows.
Machine translation engine quality shouldn’t stop you from leveraging MT to its full potential. There are many ways to approach the quality conundrum and new innovations that will enable you to go further with your translations.