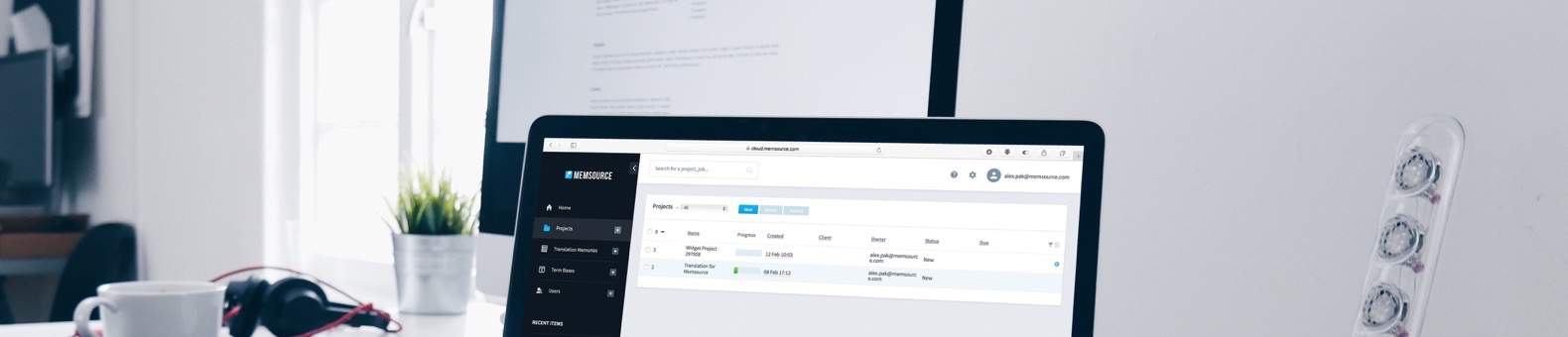
Blog
Machine Translation Report
What is the optimal MT Engine for you? Find out in the latest MT Report by Memsource.
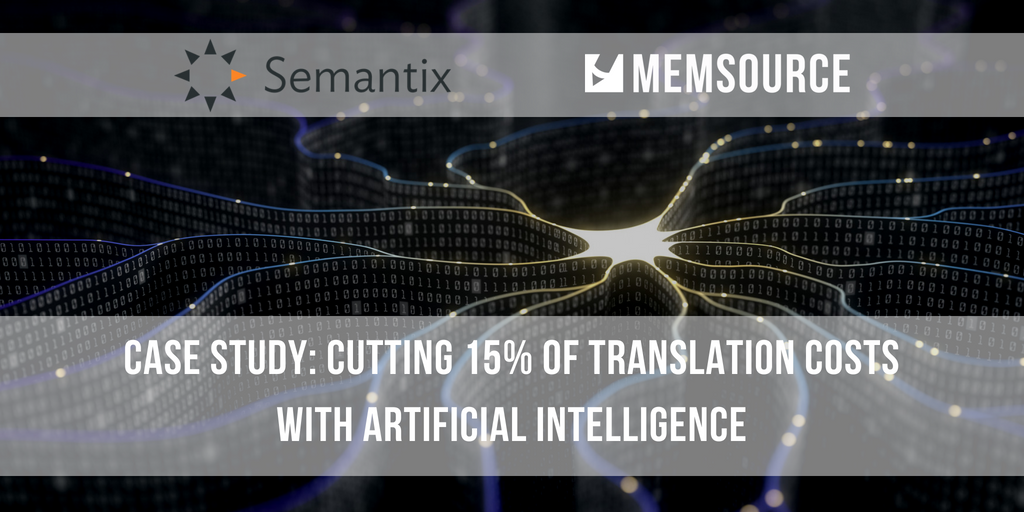
Christophe Eyraud, Solutions Architect at Semantix
In January 2018, Memsource released its first AI-powered feature, the AI-powered Non-translatables, which scans content submitted for translation and automatically detects segments that do not need to be translated. This means that translation can be done faster and at a lower cost since the volume that actually needs to be translated is reduced.
We at Semantix were one of the early adopters and I piloted this feature on real Semantix translation projects. I have summarized my findings in this case study.
Understanding the weighted word count
Before I dive into the case study, a brief and simplified explanation of how translation tools work is necessary. Translation tools include a translation memory, a database that stores previously translated segments, and let’s say that one segment corresponds to one sentence. Before you translate a file of segments, you must first analyze it.
The analysis consists of comparing the segments in the file you want to translate against the segments in the translation memory. The more translated segments you can reuse, the cheaper the translation. The analysis generates a report that indicates how many segments are identical and require no or minor editing (100% matches), how many segments are almost identical but require some editing (fuzzy matches), and how many segments do not exist in the memory and need to be translated from scratch (no matches).
The report also generates the weighted word count, which is obtained by multiplying the number of words in each match category against the discount granted for each category (100% matches are cheaper than fuzzy matches, which in turn are cheaper than no matches). The weighted word count gives you an estimate of how long and how much effort will take to translate the file.
Therefore, what matters when you translate a file is not the number of words in the file; what matters is the weighted word count you obtain after you have analyzed the file.
A promise for cost savings
How does this relate to Memsource’s new AI-powered Non-translatables feature? According to David Čaněk, CEO of Memsource, Memsource data show that 14% of all segments (which correspond to 4% of all word count in those segments) are non-translatable. These are segments that do not require translation, such as company names, product names, numbers, various codes, etc. Traditional rule-based algorithms also try to detect non-translatable segments but can only identify a limited number of them.
This is where AI comes in: not only can the artificial intelligence detect many more of the non-translatable segments, but it also gives them a score that is identical to the scores used in the analysis report mentioned above (100% non-translatable match or fuzzy non-translatable match). As we know, 100% and fuzzy matches require less editing than no matches. This means that Memsource’s new feature can help decrease the weighted word count and therefore cut the translation costs.
The case study I performed was based on real projects covering various types of documents and languages. The idea was to see what kind of segments are identified as non-translatable segments and also to compare the suggested non-translatable segments against the final translations confirmed by translators. I also used test files containing a lot of non-translatable segments to see how the feature would categorize these segments across various languages. The overall goal of the case study was not only to check whether the feature can be trusted but also, and especially, to see what benefits the feature can bring to Semantix and other Memsource users.
The Findings: testing the AI-powered Non-translatable feature
The feature detects significantly more non-translatable segments than before. In addition to numbers and tags (segments containing no letters), it now includes acronyms, alphanumeric strings, e-mail addresses, URLs, names (cities, places, people, or companies), and more.
Acronyms | Non-textual Elements |
Addresses | Numbers |
Alphanumeric Strings | Other Names (Associations, Companies) |
Codes | Phone Numbers |
Names of People | Place Names |
Dates | Plain Numbers |
Decimal Numbers | Tags |
E-mail Addresses | Times |
Measurements | URLs |
Miscellaneous | Variables |
AI-powered Non-translatables
Most of these non-translatable segments are found in texts of a technical nature (catalogs, manuals, etc.), but I also found that marketing texts, medical documents, and financial reports produced good results. Obviously, the number and type of detected non-translatable segments vary between languages since we know that not all languages are treated equally. For instance, the total coverage is higher when English is the target language (US or UK does not matter).
In some languages pairs, there were fewer 100% non-translatable matches, but many more 99% non-translatable matches. This occurs when the model is not sure: rather than suggesting a 100% non-translatable match, it applies a conservative approach and categorizes the segment as a 99% non-translatable match. One thing worth noticing is that for once, Finnish is not left behind; regarding total coverage, it is on par with French.
As explained above, 100% matches require either minor editing or none at all, and fuzzy matches always require some editing. Well, when it comes to the AI-powered non-translatable segments, 94% of the 100% non-translatable matches detected in the projects covered by the case study did not require any editing at all.
This is good news! This means that a 100% non-translatable match can be considered the same as a regular 100% match from translation memory - sometimes it needs to be edited based on the context, but most of the time it can be confirmed without changes. The other good news is that 61% of the fuzzy non-translatable segments did not require editing either. That was a nice surprise! By definition, a fuzzy match needs to be edited, however, in the case of fuzzy non-translatable matches, 6 out of 10 segments didn’t need to.
Results: Promise kept?
In summary, the AI-powered Non-translatables feature is reliable and has allowed us to lower the weighted word counts in our projects. Therefore, we are able to deliver translations faster than before and can also offer competitive quotes to our customers, who appreciate this. By using the Analysis feature that showcases the weighted word count with the AI-powered Non-translatable feature included, they can clearly see that we will not charge them for content that does not require translation. This is very important for us at Semantix – this new feature improves the relationship and increases the trust between our customers and the company.
The cost savings on the supply side are also significant. In a recent project (figure below), the actual word count was 150,000 words, and the initial weighted word count was 87,000 for the French translation. After enabling the non-translatable feature, the weighed word count dropped to 65,000. As you can see on the graph below, without non-translatable, 49% of the segments were repetitions, and 51% were not matches. After enabling the AI-powered Non-translatables, the number of no matches dropped to 36% - a decrease of 15%. We would have paid the French translator about 13,035€. After enabling the non-translatable feature, the cost dropped to 9,689€. Imagine the savings you can achieve in a multilingual project!
Translators benefit from the feature as well. Thanks to the non-translatable score, they know that some segments require less attention than others and can focus more on style and creativity and increase their productivity as a result.
Of course, the feature has to be used wisely. One should perform some tests to take into account the different factors in the equation: language combinations, source content, type of non-translatable expected, etc. Then, one should adjust the formulas and match discounts used to calculate the weighted word counts accordingly.
All of that said, the AI-powered Non-translatables feature is promising. What does it promise us? Lower purchasing costs and a better relationship with our customers.
About the Author:
Christophe Eyraud is a Solutions Architect at Semantix, the largest translation company in the Nordics. Always watching industry trends and backed by excellent relationships with technology providers, Christophe designs and implements solutions to help Semantix customers add value to their localization workflows.
About Semantix:
Semantix is the largest language company in the Nordics, providing interpreting, translation and advanced language solutions to the public sector and private corporations for more than 50 years. Semantix has a turnover of approximately SEK 900 million and operates in accordance with ISO 9001:2015. The group has offices in Sweden, Denmark, Norway and Finland and representations in China, Chile and Spain. Semantix has some 400 employees and manages a network of thousands of language specialists across the globe. Semantix is majority-owned by the private equity fund Segulah V L.P. For more information, please visit __www.semantix.eu_.